Katy, TX
Artificial intelligence (AI) has the potential to revolutionize many industries and improve people's lives in countless ways. However, AI is not immune to the biases that can exist in the data it is trained on and the people who develop it. The biases that can be found in AI systems can perpetuate and even amplify societal inequalities. Two of the most significant biases in AI systems are racial and gender biases.

Racial bias in AI can occur when the data used to train a system contains a disproportionate number of examples from one racial group over another. For example, if an AI system is trained on data that primarily contains images of white faces, it may not perform well on images of non-white faces. This can lead to a variety of negative consequences, such as difficulty in accessing certain services or being wrongly identified as a criminal suspect.
One example of this is the facial recognition technology which has been found to perform less accurately on individuals with darker skin tones, which can lead to potential wrongful identification and discrimination. Additionally, racial bias in AI can also lead to the reinforcement of stereotypes, such as the idea that people of certain races are more likely to commit crimes or be unemployed.
Gender bias in AI can occur in a similar way, when the data used to train a system contains a disproportionate number of examples of one gender over another. For example, if an AI system is trained on data that primarily contains images of men, it may not perform well on images of women. This can lead to a variety of negative consequences, such as difficulty in accessing certain services or being wrongly identified as a criminal suspect.
One example of this is the AI-powered virtual assistants which have been found to respond differently to voices based on gender, with male voices often being given more attention and taken more seriously than female voices. Additionally, gender bias in AI can also lead to the reinforcement of stereotypes, such as the idea that women are less competent than men.
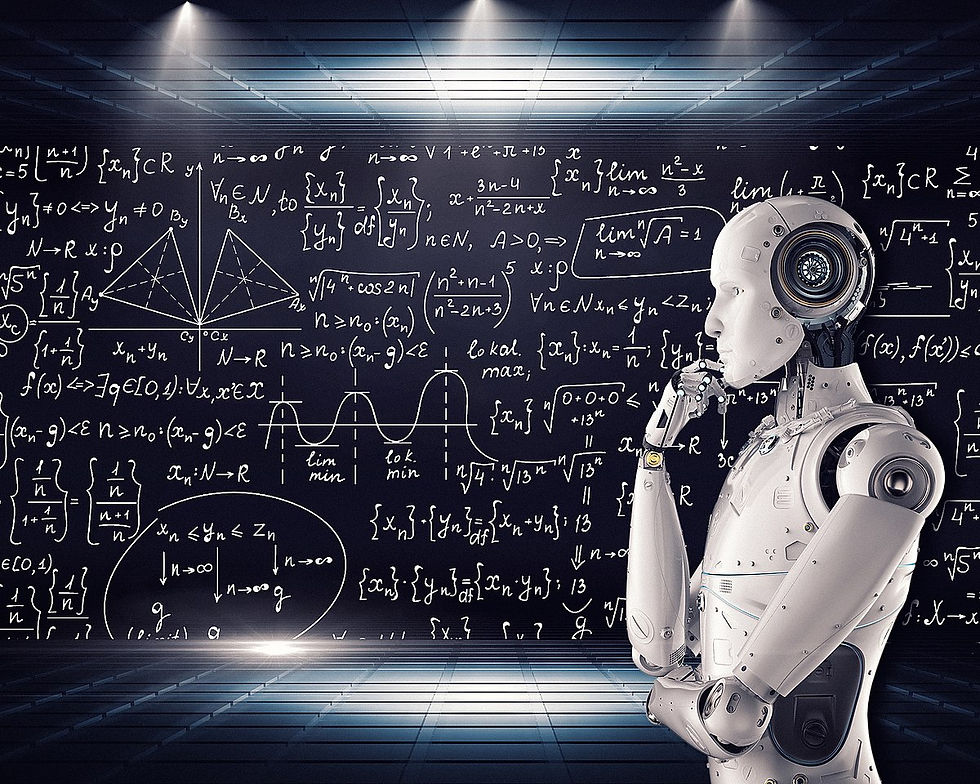
To mitigate these biases, it is crucial to ensure that the data used to train AI systems is diverse and representative of the population. It is also important to have a diverse team of developers working on AI systems to ensure that different perspectives are considered. Additionally, there are a number of techniques that can be used to reduce bias in AI systems, such as debiasing algorithms and counterfactual data augmentation. There are also organizations and initiatives like Partnership on AI, AI4All, and AI4Bias which are working towards addressing and mitigating these biases in AI. However, it is important to note that these biases can be difficult to fully eliminate, and ongoing monitoring and evaluation will be necessary to ensure that AI systems are fair and just.
In conclusion, racial and gender biases in AI can perpetuate and even amplify societal inequalities. It is important to be aware of these biases and take steps to mitigate them. This includes using diverse and representative data to train AI systems, having a diverse team of developers, and using techniques to reduce bias in AI. It is also important to remember that these biases can be difficult to fully eliminate, and ongoing monitoring and evaluation will be necessary to ensure that AI systems are fair and just.
Komentáře