By Derek Yang, edited by Ishaa
Dallas, TX
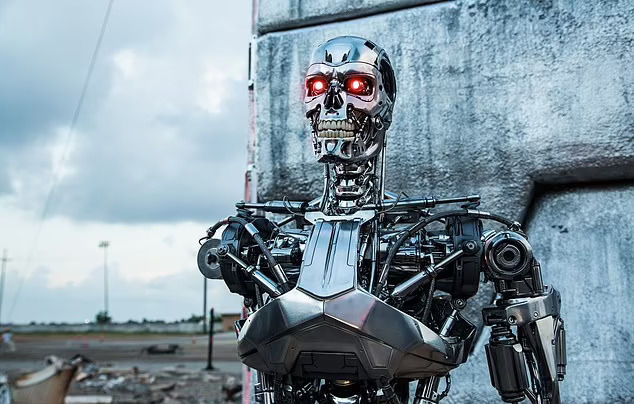
In 1872, Samuel Butler wrote his novel Erewhon, in which he first theorized human-like artificial intelligence. In his novel, the robots staged rebellions against their human masters, a trope that has been repeated time and time again throughout both fiction literature and film, such as in George Lucas’s 1977 Star Wars and Stanley Kubrick's 1968 2001: A Space Odyssey. While these scenarios of robot rebellions were never (fully) realized, another type of robot revolution occurred: the onset of robots and artificial intelligence (AI).
In the past two decades, AI and other robotics have been rapidly merged and integrated into multiple industries that the modern world relies on. While AI and robots have indeed helped expedite and streamline processes such as construction and communication, many experts are concerned with the impending labor crisis. Indeed, in many industries, robots have fully replaced humans, and will likely continue to do so. For example, some courier services traditionally run by humans on bikes or in cars have been replaced by delivery robots, drones, mini-vehicles, and full trucks. Also, many receptionists have been replaced by robots, which manage, divert, and return calls in the humans’ places.
Furthermore, AI’s widespread influence, especially those fueled by machine learning (a process in which AI “learns” about processes by recognizing patterns in massive amounts of data) is arguably having an additional negative effect, sowing distrust and misinformation among the population. By becoming increasingly integrated into social media platforms, AI can begin to erode a nation’s sense of unity and security by spreading lies. For example, AI tools such as ChatGPT and Google’s Bard have been known to spread misinformation known as “hallucinations”, reporting errors ranging from minor inaccuracies in data to generated lies. Such AI systems could be misused to spread misinformation on a massive scale if fallen into the wrong hands, such as in destabilizing a country (ex. misinformation’s role in the January 6th Capitol attacks) or fouling international relations (ex. a April deep-fake video of Ukrainian president Volodymyr Zelenskiy announcing a surrender in the Russian-Ukrainian conflict).
A third question that the modern AI takeover raises is “How can users’ information be kept private?” For AI models to be trained to recognize patterns or make decisions, models must be fed massive amounts of data. Most of this data is extremely personal and can contain information about a user’s personal life, fitness and health level, finances, relationships, location, and online activity—and often without the user’s consent.
Legal boundaries involving AI are often confusing and muddy to navigate, given the relatively novel capabilities of AI models, making it extremely difficult for consumers to fight back against bigger AI-utilizing companies.
However, despite these negative effects of AI, other experts maintain that AI will eventually be used to complement workers. In other words, they hope that workers will learn to work alongside robots to become more efficient. This position was somewhat idealistic; according to a real-world case study in McKinsey’s The State of AI in 2020, users simply did not trust the AI systems because people didn’t understand how the AI models made their decisions. This phenomenon—termed “black box”—represents a significant barrier to the widespread integration of AI.
Enter Explainable AI.
Explainable AI (XAI) refers to the tool in which the How? and Why? questions that users often have, and XAI addresses the lack of transparency associated with AI models. XAI mainly refers to the responsibility of AI developers to explain how AI models work, and this transparency can occur by breaking down processing steps.
Among the XAI techniques are prediction accuracy (an estimation of how successful the AI is on a daily basis during operation), traceability (being able to track exactly which steps the AI used to arrive at a conclusion), and decision understanding (understanding why the AI arrived at the conclusion). Decision understanding is arguably the most important factor in increasing engagement with AI, as understanding the rationale of the AI helps to build feelings of trust and reliability.
Such explanations of how AI models operate can help non-technical audiences gain a more thorough understanding of how AI works, helping to clarify questions and misconceptions. By explaining AI to wider audiences, XAI also helps to make AI usage and fluency more accessible to diverse audiences, especially those in socioeconomically disadvantaged communities, which in turn has multiple implications in improving access to education and productive services. Explainable AI can also increase engagement with company services to generate greater amounts of profit.
For example, XAI can be used in healthcare settings to integrate AI smoothly at the bedside by accelerating diagnostics, analyzing images (and providing healthcare professionals with more standardized diagnoses), and streamlining the pharmaceutical approval process. In the financial sector, XAI can be leveraged to transform traditionally “black-boxed” services like loan and credit approval processes more transparent, which mitigates the potential for complaints and other issues. Finally, XAI can also be used in the legal field to test for potential biases in machine learning datasets and AI models, helping make the modern criminal justice system more equitable and fair.
There is no doubt that AI will become an integral part of our daily lives. While it has replaced many blue-collar jobs (and certainly will continue to), AI also has the potential to serve us in much greater ways, streamlining economic and industrial processes. With explainable AI widely implemented, thereby fostering trust and transparency in AI model development, AI integration will become smoother and widespread.
References
Tell us what interests you! Drop suggestions to read about your favorite STEM topics!
תגובות